DECISION MAKING – SUPPLY CHAIN
Parsimony allows us to reduce the amount of data needed for learning. AI can then be deployed quickly.
Instead of collecting data for years, a week might be enough. Instead of collecting data for weeks, an hour might be enough…
This asset is of capital importance in a constantly changing world. Indeed, the data collected over too long time quickly becomes obsolete.
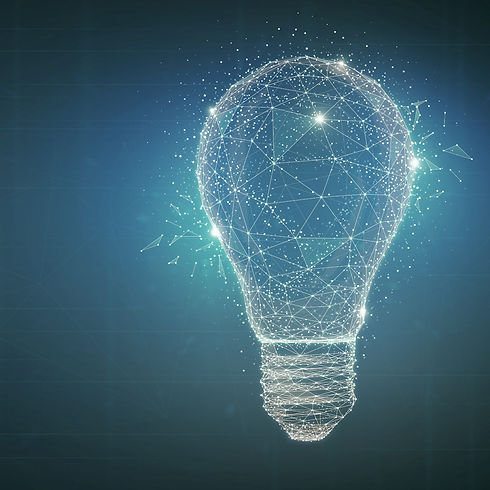
OUR SUCCESS STORIES
Distributed gas
quality forecasting
Aircraft tires
wear prediction

Satellite image
processing
Real time beam forming of array antenna
Wind farm
production prediction
Supply chain
Context
-
The aim is to predict when customers will send tires back for retreading or require replacement products.
-
Previously the company had no accurate system to do this and was based on feel not statistical analysis.
Goal of the study
Predict the number and dates of tires returned over the next 12 months.
Challenge
Small data context as each customer uses their tires differently and its hard to extrapolate the data
Methodology
-
Compress the input data, compress the output data, create parsimonious model in the compressed space.
-
End to end learning.
Supply chain
Objective
Aim to predict client demand and to schedule just in time production of the good/service.
Why NeurEco
Client demand is dynamic and constantly changing. Conventional modeling technology is not able to accurately model this and changing production patterns. NeurEco is custom designed to do this.
Benefits to the client
-
Clients can schedule manufacturing in an efficient manner allowing the client to minimize the time inventory spent in warehouses and allowing for a larger profitability.
-
Clients can use the data for new product releases and to easily exceed their clients expectations.